As COVID-19 Accelerates, Governments Must Harness Mobile Data to Stop Spread
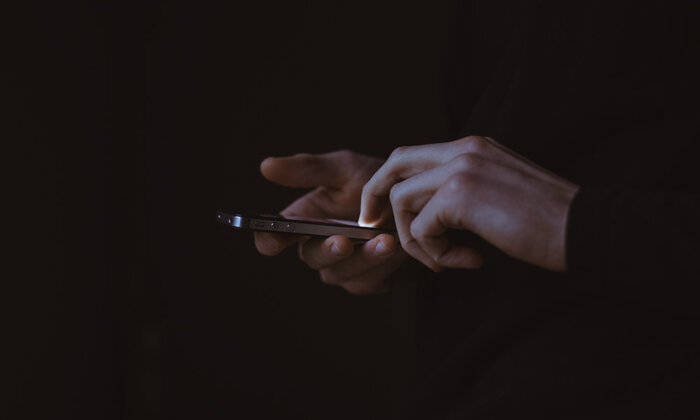
Last week, Israel’s internal security service agency began sending text messages to its citizens who may have been exposed to Covid-19. “According to an epidemiological investigation, you were near someone sick with the coronavirus,” the text began, one source told NPR. “You must immediately isolate at home [for 14 days] to protect your relatives and the public.”
While some privacy advocates have objected to such surveillance, we believe other democratic governments should follow Israel’s lead — and quickly. Using trajectory data, or location data, from smartphones to speed up “contact tracing” could help contain SARS-CoV2, the fast-growing novel coronavirus responsible for the global Covid-19 pandemic.
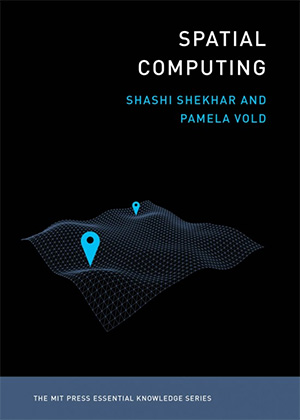
Contact tracing has been used by public health experts for decades to control spread during the initial stage of a communicable disease. It was effective in fights against HIV, measles, SARS-CoV (the 2003 SARS outbreak), and even, in places like Singapore, SARS-CoV2. In 2003, it significantly reduced the transmission risk of SARS in Hong Kong. And before that, it played a pivotal role in the eradication of smallpox by identifying and isolating infected persons and immunizing at-risk contacts and surrounding communities. Traditional contact tracing interviews take place with infected persons to gather data about their movement and people they have been in close contact with. But this manual process is too slow and labor-intensive to effectively stop fast-developing outbreaks, such as SARS-CoV2. Smartphone trajectory data can instead be used to greatly speed up — and scale up — contact tracing for large and rapidly evolving outbreaks of infectious diseases.
Here’s how it might work: One could start with the registry of people who have tested positive for SARS-CoV2 and estimate the onset of the infection which may precede the test by days. This information could then be used to create an infected trajectory dataset, which could be checked against the trajectory of all smartphones to identify the phones that were spatially close for a substantial duration. From there, the spatial distance and exposure time could be processed via an infectious disease transmission model to assess the risk of transmission and the need to notify the exposed. Once the contacts are determined, public safety communication technology such as Reverse 911 could be used to deliver medical advice to those who have potentially been exposed. If smartphone trajectories of non-infected individuals need to be excluded for privacy reasons, the locations and times of potential exposure could be publicly shared without divulging patient names or sensitive medical information.
Not only are these strategies more efficient than manual contact tracing, they also improve the quality of travel history data when infected persons cannot recall all the places they recently visited. Further, they allow for a more thoughtful choice of distance thresholds to assess exposure, accounting for wind-speed and contact durations. Moreover, they reduce spurious warnings to community members who were not in contact with infected persons, which could help ease anxiety.
Smartphone-location-based contact tracing could not only save lives now, but also provide valuable information to public health researchers when it comes to containing future waves of SARS-Cov2 and other communicable diseases.
Mobile-phone location data analysis is an example of spatial computing, which is already used widely to map and track diseases and their hotspots. Billions of people around the globe use various applications of spatial computing daily — by using smartphone apps for navigation, ride-sharing, and social media check-ins, for example.
Environmental scientists use GPS-trajectory data to understand migration routes, habitats, and social structures of endangered species. Smartphone trajectories have the potential to improve the efficiency of transportation, which is a major source of greenhouse gases, and to reduce associated emissions and energy use. In addition, transportation planners collect trajectory data via travel-survey apps and leverage it not only to plan future highways but also to facilitate car-pooling. Travel survey apps are considered an improvement on traditional paper-based survey methods as they lower participation burden and improve data quality. Further, the public health community uses geo-simulations of epidemiological models of infectious diseases and interventions across alternative scenarios to identify the best ways to control disease spread.
Use of trajectory data for contact tracing in democratic societies must of course consider the issue of data privacy since smartphone data can reveal sensitive information. But there are ways to do this without giving up individual privacy, as MIT is doing with its open-source Safe Paths software, which securely logs your location and notifies you if you’re at risk.
Wireless Emergency Alerts already deliver messages to individuals at risk of contact with a life-threatening hazard such as a hurricane, tornado, flash flood, tsunami, or dust storm. This is done without an explicit request from the smartphone owner. Our society also has preexisting norms for sharing HIPAA-protected infection information to vulnerable contacts. For example, all states and the District of Columbia have HIV registries and partner-notification laws to facilitate communication of a positive HIV test to at-risk parties, such as sex or needle-sharing partner(s). Further, recent guidance issued by the U.S. Department of Health and Human Services clarifies that disclosure of protected health information to public health authorities, whether local, state, or federal, and to persons at risk, is permissible without individual authorization.
This is not the time for governments to drag their feet. Smartphone-location-based contact tracing could not only save lives now, but also provide valuable information to public health researchers to improve the understanding of epidemiology and improve intervention methods when it comes to containing future waves of SARS-Cov2 and other communicable diseases. In these times of crises, we need to make use of all the tools available to us.
Shashi Shekhar is McKnight Distinguished Professor in the Department of Computer Science and Engineering at the University of Minnesota and a co-author of “Spatial Computing.” Apurv Hirsh Shekhar is an MD candidate at Yale School of Medicine and an honors graduate of Johns Hopkins University, where he studied Public Health and Chemical & Biomolecular Engineering.