What Internet Search Patterns Can Teach Us About Coping

Fifty years ago, renowned psychiatrist Elisabeth Kübler-Ross published a book that would change the way the medical community cared for the terminally ill. “On Death and Dying” shattered the American taboo of talking about death and laid the foundation for a five-stage grieving model that is still widely accepted today.
Medical practitioners who are asked about the Kübler-Ross model agree that while the five stages — denial, anger, bargaining, depression, and acceptance — may not always unfold in that exact order, the model remains, broadly, correct, and can be applied to other kinds of losses, including those associated with cancer. But how do they know this, beyond their personal, anecdotal, experience? A search of the scientific literature reveals that about 3,000 scientific papers mention this model, and while some substantiate it through qualitative data, the quantitative literature remains scarce. As a data scientist focused on using data from the internet to improve health, I wondered what one would have to do to remedy this.
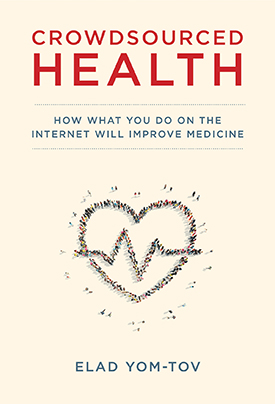
One approach might be to have an experimenter sit outside the office of an oncologist and wait for people who emerge, having been told that they have been diagnosed with cancer. The student would ask each patient — whose life had just been turned upside down — to answer a set of questions about their state of mind, and then to complete the same survey once every few hours so that their state of mind could be tracked as they passed through the stages.
But even less challenging psychological studies have found it difficult to recruit participants. One study contacted surviving relatives of the deceased, whose names were obtained from newspaper obituaries, only to be turned away by nearly 50 percent of them. In another, researchers tried to get the parents of children newly diagnosed with cancer to take part in six sessions designed to assist them in coping with the child’s disease. As the researchers reported, many parents were unwilling or unable to do this because they were “feeling overwhelmed.” They had bigger problems on their minds than helping to validate a psychological model, even if it was for their own benefit.
What if we could identify people, I wondered, who had just been diagnosed with cancer and who were searching the internet for information about it? By classifying the webpages they read or the queries they made as they searched for information, we might see whether they indeed passed through the five stages of grief. Together with blood cancer specialist Yishai Ofran and data scientist Dan Pelleg, I set out to do just that.
Identifying people who had been diagnosed with cancer, or whose family members or friends had been diagnosed, was relatively simple. We looked for people who suddenly developed an intense interest in a specific type of cancer. For example, people who, suddenly, began searching for information about colon cancer, and kept doing so for a number of days. After narrowing our list down from 232,681 anonymous users to a smaller group of 50,117 users that had the most interest in this topic, we discovered that about 6,000 webpages were read by many of the people in the smaller cohort. Our next step was to ask a few psychologists, “What would a person in the denial stage (or the depression stage) read?” This, it turns out, is a difficult question — so difficult that we could not get a consensus among the psychologists on how to identify the stage people were in according to the pages they were reading.
The problem, essentially, was that it was impossible to decide which pages were read when people were in the different stages (assuming that, indeed, these stages exist). But perhaps we could divide these pages into more easily identifiable categories, for example, according to whether they dealt with treatments of cancer, with support networks, or with diagnosis. We had to change gears.
Because the sheer volume of the data would require several weeks of work by a single person, we turned to a tool that is widely used by businesses and researchers, though rarely discussed: Amazon’s Mechanical Turk. Deriving its name from a chess-playing machine that purportedly could play at a high level against a human opponent but was later revealed to house a human who slyly moved the pieces, Mechanical Turk is a market in which the product traded is human effort. Say, for example, you want to extract text from a large number of pictures showing street signs; using the service, you could crowdsource the work for as little as a few pennies per task. Because even a human makes mistakes, the same task is usually sent to several people, after which the most common answer given by them is used. This is a cheap and effective way to solve tasks that require intensive human effort.
According to our data, the kind of information someone needs during the first few days after that person or a close loved one has been diagnosed with cancer changes rapidly.
Using Mechanical Turk, we divided our 6,000 webpages into eleven general categories within a few days. These were categories that were easy for a layperson to decide on, including a description of symptoms, being the page of a support group, or a list of treatments for cancer. The next step required linking our 11 categories to hidden stages reflecting people’s underlying mental state. For this, we employed a Hidden Markov model, a mathematical tool that helps to uncover hidden layers from observed data. Think of it like this: You’re in a windowless building and cannot see whether it is rainy or sunny outside; you might deduce that it is raining when you see people entering the building wearing wet clothes. The hidden state here is the weather; the observed data is the clothing. Though Hidden Markov models may seem abstract, they have found uses in many areas, including speech recognition, bioinformatics, and cryptanalysis. In our case, they provided a solution to our mapping problem.
Let us assume that people pass through some distinct mental stages during their grief, and that in each stage they encounter different information needs. These needs are apparent from the webpages they read. In analogy to the Hidden Markov Model, the hidden states correspond to the mental stages between which people transition; the visible states are the subjects of the pages that people read, as classified by the Mechanical Turk workers. All the visible states may appear when people are in different hidden states, but the probability that each state will appear is different.
One drawback of using Hidden Markov Models is that it is difficult to associate a specific hidden state with one of Kübler-Ross’s five stages. But if the data support the use of a model with distinct transitions, such an association may not be overly important.
Using search-engine queries submitted to the Yahoo engine during a six month period by 20,808 people who visited pages of more than one category, we tried to fit the best Hidden Markov Model to the pages people read as results of their queries. Once we saw that a Hidden Markov Model with five hidden states was indeed a good way to describe the search behaviors we had observed, we built separate models for people who asked about acute forms of cancer and for people who asked about more chronic cancers, the two groups being defined according to rates of survival.
The two models turn out to be very different. The model that describes the behavior of people searching for information on acute cancers tends to see people remaining in the first three stages, and the behavior of such people is more string-like than that of people searching for information on chronic cancers (that is, they are less likely to go back to earlier stages). People asking about chronic cancers, on the other hand, are more likely to fall into the latter two stages and tend to stay in them longer than those suffering from acute cancers. The stages also differ in the information people seek: in cases of chronic cancers social support is an important topic, whereas in cases of acute cancers treatment options feature prominently.
We had one more phenomenon to investigate, though it did not bear on the five stages of grief.
At the time, Yahoo ran one of the most extensive social networks on the internet, a network that allowed people to chat via text or voice. We overlaid this network on the search data and examined how the searches of friends of people diagnosed with cancer looked when they searched for information about cancer.
A first sign that something interesting happens between friends is our observation that if two friends on Yahoo’s network searched for information on a specific type of cancer, they were more than twice as likely to search for information on the same type of cancer as would be expected to occur by chance. We again divided the population into two groups according to the severity of the type of cancer they were seeking information about. In cases of aggressive cancer, both people in a pair searched for about twelve days, and the second person began to search nine days after the first person began to do so. In cases of less aggressive cancers, the first person to begin searching also searched for about twelve days; the friend began searching fifteen days later and searched for only five days.
The first of a pair of friends to begin searching was also more likely than the second to be interested in treatments for cancer and in general information about the disease. The second person to begin searching was more likely to look for pages related to the causes of cancer and for those promising help with social support.
People who have been diagnosed with acute cancers have less time to search for information because they need to begin treatment; their friends do the searching for them.
We believe that the first of a pair of friends to begin searching is either the patient or a close family member. Such people begin searching once they know they have cancer, or are informed of a strong suspicion to that effect. Friends and more distant relatives begin searching later. People who have been diagnosed with acute cancers have less time to search for information because they need to begin treatment; their friends do the searching for them, as is evident from the longer search periods of the second searchers. In cases of less acute cancers, the patient can afford to search for information for a longer time, and after a relatively short search, a friend may decide that the disease is not as severe as had been thought.
Why are these findings interesting, beyond giving some support to the five-stages-of-grief model? I believe that the data provide an important and otherwise difficult to obtain insight into the critical period that follows diagnosis. During that short period (which our data suggest is well under two weeks), people are thirsty for information, but their information needs change rapidly as they transition between stages. The medical community should recognize this, and should provide information in a way that is aligned with patients’ needs, emphasizing some aspects of the information more than others. Moreover, it is important to understand what stage a patient is in, perhaps by asking the patient some guiding questions or listening to his or her concerns (even non-medical concerns) just to detect what information they are seeking.
According to our data, the kind of information someone needs during the first few days after that person or a close loved one has been diagnosed with cancer changes rapidly. It changes, most probably, for several reasons: First, the way the person grapples with the new condition changes as they go through the five stages of grief. Second, as someone learns more about their disease, they may want to gain an even deeper understanding of its specific facets, or of particular treatment options. Therefore, after a doctor informs a patient of a cancer diagnosis, it may well be more beneficial for the doctor to schedule several short meetings over the following days to discuss the disease and the options for treatment, rather than for the doctor to give the patient all the information at once.
Information providers online should also tailor their information according to patients’ needs and interests. For example, the pages devoted to specific cancers on many highly visited medical websites, including the Mayo Clinic’s, begin with symptoms, causes, and risk factors. However, a person who already has been diagnosed with cancer will have very little use for these materials. He already knows that he has the disease. Websites should cater to people already diagnosed with a disease in a way that takes their mental states into account. As we have learned from our data, people with different mental states require different information, and their mental state changes rapidly in the first few days after diagnosis.
Of course, our data are far from perfect: Our evidence on the medical status of our users is circumstantial. While this is an important feature to preserve the privacy of our users, it also means that we may be missing many people who are ill but use search engines in a way that does not allow us to identify them. We may also be mislabeling people who we infer are sick with the condition we are analyzing. Similarly, our measurements of mental states are implicit. Thus, research using internet data should be considered a complementary approach to medical research, not one which replaces more traditional methods.
As I argue in my book “Crowdsourced Health,” information collected online can benefit humanity without sacrificing individual privacy. Already, searches have aided researchers in tracking the side effects of prescription drugs and identifying some of the causes of and risk factors for anorexia. In the three short years since the book was published, our understanding of people and their health, as evident from internet data, has greatly advanced. We can measure people’s anxiety through their interaction with search engines, for instance, and have shown how this mental state affects the way they collect information. Perhaps most exciting is the fact that we are able to detect several types of cancer (as well as other medical conditions) from people’s search engine queries sometimes well before they know they have the condition. Half a century ago, “On Death and Dying” had a profound effect on the way doctors attended to end-of-life issues. Today’s tools open up yet new ways to understand people’s needs at a time of great mental burden — tools that, if used prudently, could usher in the next generation of care.
Elad Yom-Tov is a Principal Researcher at Microsoft Research Israel. He is the author of “Crowdsourced Health: How What You Do on the Internet Will Improve Medicine.“